Additionally, the sheer number of robots in Mann vs Machine makes a Puff 'n Sting strategy with the Axtinguisher less effective, as burning down all of the robots in a group with a flamethrower is quicker than meleeing them one by one. Secondary and Melee Weapons. The Gas Passer is a recommended secondary choice in Mann Vs Machine. Never Get Attached! Never ever pick a favorite slot machine! If you get used to playing on one.
- Progressive Slot Machine Strategy
- Machine Strategy Definition
- Dokkan Punch Machine Strategy
- Machine Learning Strategy
We all believe that slot machines, one of the most popular casino games, are purely based on luck and nothing else. While this is quite near to truth there are still some ways using which you can improve your chances at winning that eye-catching jackpot on the slot machine. Today we're going to touch on the 5 reel online slot machine strategies you never knew existed. They're fairly basic and really easy to understand.
Determine your bankroll before you get to the casino. This is the most important tip I can give you.
5. Have a Betting Pattern – Have Control over Your Bankroll
Let us start off by establishing a certain pattern for your bets. Consider starting with the lowest available bet. When you have lost several spins or hands, you should increase it by one; if you win, decrease it by one. While this strategy does not guarantee winning, it is a good way to have control over your bankroll and not to lose everything at once. Keep in mind that in the end it is the bankroll that is important.
4. Jackpots – Bigger Isn't Always Better
In many casinos, the bigger 6-or-7-figure jackpots seem almost unattainable. On the other hand, you'll see the smaller jackpot slots pay out more frequently. On huge progressive jackpot slot machines, a good chunk of each bet goes to feed the dedicated jackpot, but the potential payout is also ridiculously large. So if you want to win more for less playing slot machine with larger jackpots is also an option.
3. Know the Payouts!
Slot machines that are based on reels have variant programming, meaning that the payout percentage may vary above and below its standard payout percentage. For example, if you are playing on a machine with a payout rate of 95% at times it might pay out 93% or 97%.
2. Weighing Your Chances
Always think of the probability and what your chances of winning are. For example, let's say the machine you are playing on has 3 reels, each with 10 stops and a symbol on each of them is for the jackpot; If this is the case, three jackpot symbols would line up, on the average, once every 10310310 pulls, or 1,000 pulls. Weighing your chances, gives you more control over the game play.
1. Never Get Attached!
Never ever pick a favorite slot machine! If you get used to playing on one and the same machine each time you visit a casino because it treats you well, that might cause you some trouble. If it starts treating you bad, you might hesitate on giving it up and changing slot machines frequently is one of the primary strategies for gamblers. The basic rule is to keep going if you are breaking even and change machines if you are not.
Of course, the majority still depends on luck and probability, but generating a certain strategy can truly make some difference on the final outcome and play in your favor! Who owns mybookie.
Gemma Sykes
Gemma is not only a great game player who enjoys casino halls, she is also a great jazz dancer. She has a very keen interest in the way things work, her curiosity got her a job on online gambling industry as a writer. Always looking for new and fun ways to do things and still have time for the spotlight.
Latest posts by Gemma Sykes (see all)
Join Transform 2021 for the most important themes in enterprise AI & Data. Learn more.
Most companies are struggling to develop working artificial intelligence strategies, according to a new survey by cloud services provider Rackspace Technology. The survey, which includes 1,870 organizations in a variety of industries, including manufacturing, finance, retail, government, and healthcare, shows that only 20 percent of companies have mature AI/machine learning initiatives. The rest are still trying to figure out how to make it work.
There's no questioning the promises of machine learning in nearly every sector. Lower costs, improved precision, better customer experience, and new features are some of the benefits of applying machine learning models to real-world applications. But machine learning is not a magic wand. And as many organizations and companies are learning, before you can apply the power of machine learning to your business and operations, you must overcome several barriers.
Three key challenges companies face when integrating AI technologies into their operations are in the areas of skills, data, and strategy, and Rackspace's survey paints a clear picture of why most machine learning strategies fail.
Machine learning is about data
Machine learning models live on compute resources and data. Thanks to a variety of cloud computing platforms, access to the hardware needed to train and run AI models has become much more accessible and affordable.
But data continues to remain a major hurdle in different stages of planning and adopting an AI strategy. Thirty-four percent of the respondents in the Rackspace survey stated poor data quality as the main reason for the failure of machine learning research and development, and another 31 percent said they lacked production-ready data.
This highlights one of the main hurdles when applying machine learning techniques to real-world problems. While the AI research community has access to many public datasets for training and testing their latest machine learning technologies, when it comes to applying those technologies to real applications, getting access to quality data is not easy. This is especially true in industrial, health, and government sectors, where data is often scarce or subject to strict regulations.
Data problems crop up again when machine learning initiatives move from the research to the production phase. Data quality remains the top barrier when it comes to using machine learning to extract valuable insights. Data engineering problems also pose a significant problem, such as data being siloed, lack of talent to connect disparate data sources, and not being fast enough to process data in a meaningful way.
Above: Data accounts for most key problems in gaining actionable insights from machine learning models (Source: Rackspace Technology)
Progressive Slot Machine Strategy
Both startups and established companies suffer from data problems, though scale seems to be the key differentiator between the two, according to Jeff DeVerter, CTO of Rackspace Technology. 'Startups tend to be constrained with not all the right resources to implement a quality data pipeline and consistently managing it over time,' DeVerter said to TechTalks in written comments. 'Enterprises usually have scale on their side and with that comes the rigor that's required.'
The best way companies can prepare for the data challenges of AI strategies is to do a full evaluation of their data infrastructure. Eliminating silos should be a key priority in every machine learning initiative. Companies should also have the right procedures for cleaning their data to improve the accuracy and performance of their machine learning models.
AI talent is still in high demand
The second area of struggle for most companies is access to machine learning and data science talent. According to Rackspace's survey, lack of in-house expertise was the second biggest driver of failure in machine learning R&D initiatives. Lack of skill and difficulty in hiring was also a key barrier in adopting AI technologies.
Above: Many companies struggle with acquiring the talent to implement their AI strategies (Source: Rackspace Technology)
With machine learning and deep learning having reached mainstream use in production environments only recently, many smaller companies don't have data scientists and machine learning engineers who can develop AI models.

And the average salary of data scientists and machine learning engineers matches those of experienced software engineers, which makes it difficult for many companies to put together a talented team that can lead its AI initiative.
While the shortage of machine learning and data science talent is well known, one thing that has gone mostly unnoticed is the need for more data engineers, the people who set up, maintain, and update databases, data warehouses, and data lakes. Per Rackspace's figures, many initiatives fail because companies don't have the talent to adapt their data infrastructure for machine learning purposes. Breaking down silos, migrating to cloud, setting up Hadoop clusters, and creating hybrid systems that can leverage the power of different platforms are some areas where companies are sorely lacking. And these shortcomings prevent them from making company-wide deployments of machine learning initiatives.
With the development of new machine learning and data science tools, the talent problem has become less intense. Google, Microsoft, and Amazon have launched platforms that make it easier to develop machine learning models. An example is Microsoft's Azure Machine Learning service, which provides a visual interface with drag-and-drop components and makes it easier to create ML models without coding. Another example is Google's AutoML, which automates the tedious process of hyperparameter tuning. While these tools are not a replacement for machine learning talent, they lower the barrier for people who want to enter the field and will enable many companies to reskill their tech talent for these growing fields.
'Lack of in-house data science talent is not the barrier it once was now that more of these services are able to use their own ML to help in this regard as well consulting firms having these talents on-staff,' DeVerter said.
Other developments in the field are the evolution of cloud storage and analysis platforms, which have considerably reduced the complexity of creating the seamless data infrastructures needed to create and run AI systems. An example is Google's BigQuery, a cloud-based data warehouse that can run queries across vast amounts of data stored in various sources with minimal effort.
We're also seeing growing compatibility and integration capabilities in machine learning tools, which will make it much easier for organizations to integrate ML tools into their existing software and data ecosystem.
Before entering an AI initiative, every organization must make a full evaluation of in-house talent, available tools, and integration possibilities. Knowing how much you can rely on your own engineers and how much it will cost you to hire talent will be a defining factor in the success or failure of your machine learning initiatives. Also, consider whether re-skilling is a possible course of action. If you can upskill your engineers to take on data science and machine learning projects, you will be better off in the long run.
Outsourcing AI talent
Another trend that has seen growth in recent years is the outsourcing of AI initiatives. Only 38 percent of the Rackspace survey respondents relied on in-house talent to develop AI applications. The rest were either fully outsourcing their AI projects or employing a combination of in-house and outsourced talent.
Above: Most companies rely on outside talent to plan and implement their AI initiatives (Source: Rackspace Technology)
Machine Strategy Definition
There are now several companies that specialize in developing and implementing AI strategies. An example is C3.ai, an AI solutions provider that specializes in several industries. C3.ai provides AI tools on top of existing cloud providers such as Amazon, Microsoft, and Google. The company also provides AI consultancy and expertise to take customers step by step through the strategizing and implementation phases.
According to the Rackspace report: 'A mature provider can bring everything from strategy to implementation to maintenance and support over time. Strategy can sidestep the areas where AI and machine learning efforts may lose momentum or get lost in complexity. Hands-on experts can also spare organizations from the messy work of cleanup and maintenance. Such expertise, taken together, can make all the difference in finally achieving success.'
It is worth noting, however, that fully turning over an organization's AI strategy to outside providers can be a double-edged sword. A successful strategy requires close cooperation between AI specialists and subject matter experts from the company that is implementing the strategy.
'This is very similar to companies who move to a DevOps development methodology and attempt to outsource the entirety of the development. DevOps requires a close partnership between the developers, business analysts, and others in the business,' DeVerter said. 'In the same way, AI projects require strategy and technical expertise — but also require a tight partnership with the business as well as leadership.'
Outsourcing AI talent must be done meticulously. While it can speed up the process of developing and implementing an AI strategy, you must make sure that your experts are fully involved in the process. Ideally, you should be able to develop your own in-house team of data scientists and machine learning engineers as you work with outside experts.
How do you evaluate your AI strategy?
Finally, another area that is causing much pain for companies embarking on an AI journey is forecasting the outcome and value of AI strategies. Given the application of machine learning being new to many areas, it's hard to know in advance how long an AI strategy will take to plan and implement and what the return on investment will be. This in turn makes it difficult for innovators in organizations to get others on board when it comes to garnering support for AI initiatives.
Of the respondents of the Rackspace survey, 18 percent believed that a lack of clear business case was the main barrier to adopting AI strategies. Lack of commitment from executives was also among the top barriers. Lack of use cases and commitment from senior management show up again among the top challenges in the machine learning journey.
'AI often wanders around as a solution looking for a problem within organizations. I believe this is one of the greatest impediments to its wide-scale adoption within organizations,' DeVerter said. 'As AI practitioners can demonstrate practical examples of how AI can benefit their specific company — leadership will further fund those activities. Like any business venture — leadership needs to know how it will either help them save or make money.'
Evaluating the outcome of AI initiatives is very difficult. According to the survey, the top-two key performance indicators (KPI) for measuring the success of AI initiatives were profit margins and revenue growth. Understandably, this focus on quick profits is partly due to the high costs of AI initiatives. According to the Rackspace survey, organizations spend a yearly average of $1.06 million on AI initiatives.
But while a good AI initiative should result in revenue growth and lower costs, in many cases, the long-term value of machine learning is the development of new use cases and products.
'Short-term financial gains can be myopic if they aren't paired with a long-term strategy that can be funded by those short-term gains,' DeVerter said.
If you're in charge of the AI initiative in your organization, make sure to clearly lay out the use cases, the costs, and the benefits of your AI strategy. Decision-makers should have a clear picture of what their company will be embarking on. They should understand the short-term benefits of investing in AI, but they should also know what they will gain in the long run.
Ben Dickson is a software engineer and the founder of TechTalks. He writes about technology, business, and politics. This post was originally published here as a series exploring the business of artificial intelligence.
This story originally appeared on Bdtechtalks.com. Copyright 2021
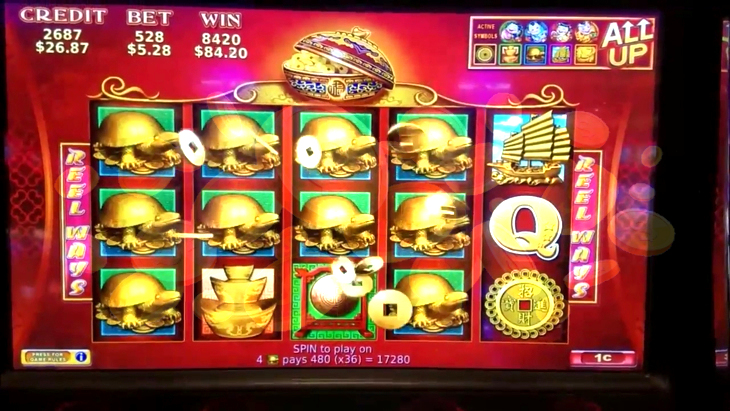
VentureBeat
VentureBeat's mission is to be a digital town square for technical decision-makers to gain knowledge about transformative technology and transact.Our site delivers essential information on data technologies and strategies to guide you as you lead your organizations. We invite you to become a member of our community, to access:Dokkan Punch Machine Strategy
- up-to-date information on the subjects of interest to you
- our newsletters
- gated thought-leader content and discounted access to our prized events, such as Transform 2021: Learn More
- networking features, and more
And the average salary of data scientists and machine learning engineers matches those of experienced software engineers, which makes it difficult for many companies to put together a talented team that can lead its AI initiative.
While the shortage of machine learning and data science talent is well known, one thing that has gone mostly unnoticed is the need for more data engineers, the people who set up, maintain, and update databases, data warehouses, and data lakes. Per Rackspace's figures, many initiatives fail because companies don't have the talent to adapt their data infrastructure for machine learning purposes. Breaking down silos, migrating to cloud, setting up Hadoop clusters, and creating hybrid systems that can leverage the power of different platforms are some areas where companies are sorely lacking. And these shortcomings prevent them from making company-wide deployments of machine learning initiatives.
With the development of new machine learning and data science tools, the talent problem has become less intense. Google, Microsoft, and Amazon have launched platforms that make it easier to develop machine learning models. An example is Microsoft's Azure Machine Learning service, which provides a visual interface with drag-and-drop components and makes it easier to create ML models without coding. Another example is Google's AutoML, which automates the tedious process of hyperparameter tuning. While these tools are not a replacement for machine learning talent, they lower the barrier for people who want to enter the field and will enable many companies to reskill their tech talent for these growing fields.
'Lack of in-house data science talent is not the barrier it once was now that more of these services are able to use their own ML to help in this regard as well consulting firms having these talents on-staff,' DeVerter said.
Other developments in the field are the evolution of cloud storage and analysis platforms, which have considerably reduced the complexity of creating the seamless data infrastructures needed to create and run AI systems. An example is Google's BigQuery, a cloud-based data warehouse that can run queries across vast amounts of data stored in various sources with minimal effort.
We're also seeing growing compatibility and integration capabilities in machine learning tools, which will make it much easier for organizations to integrate ML tools into their existing software and data ecosystem.
Before entering an AI initiative, every organization must make a full evaluation of in-house talent, available tools, and integration possibilities. Knowing how much you can rely on your own engineers and how much it will cost you to hire talent will be a defining factor in the success or failure of your machine learning initiatives. Also, consider whether re-skilling is a possible course of action. If you can upskill your engineers to take on data science and machine learning projects, you will be better off in the long run.
Outsourcing AI talent
Another trend that has seen growth in recent years is the outsourcing of AI initiatives. Only 38 percent of the Rackspace survey respondents relied on in-house talent to develop AI applications. The rest were either fully outsourcing their AI projects or employing a combination of in-house and outsourced talent.
Above: Most companies rely on outside talent to plan and implement their AI initiatives (Source: Rackspace Technology)
Machine Strategy Definition
There are now several companies that specialize in developing and implementing AI strategies. An example is C3.ai, an AI solutions provider that specializes in several industries. C3.ai provides AI tools on top of existing cloud providers such as Amazon, Microsoft, and Google. The company also provides AI consultancy and expertise to take customers step by step through the strategizing and implementation phases.
According to the Rackspace report: 'A mature provider can bring everything from strategy to implementation to maintenance and support over time. Strategy can sidestep the areas where AI and machine learning efforts may lose momentum or get lost in complexity. Hands-on experts can also spare organizations from the messy work of cleanup and maintenance. Such expertise, taken together, can make all the difference in finally achieving success.'
It is worth noting, however, that fully turning over an organization's AI strategy to outside providers can be a double-edged sword. A successful strategy requires close cooperation between AI specialists and subject matter experts from the company that is implementing the strategy.
'This is very similar to companies who move to a DevOps development methodology and attempt to outsource the entirety of the development. DevOps requires a close partnership between the developers, business analysts, and others in the business,' DeVerter said. 'In the same way, AI projects require strategy and technical expertise — but also require a tight partnership with the business as well as leadership.'
Outsourcing AI talent must be done meticulously. While it can speed up the process of developing and implementing an AI strategy, you must make sure that your experts are fully involved in the process. Ideally, you should be able to develop your own in-house team of data scientists and machine learning engineers as you work with outside experts.
How do you evaluate your AI strategy?
Finally, another area that is causing much pain for companies embarking on an AI journey is forecasting the outcome and value of AI strategies. Given the application of machine learning being new to many areas, it's hard to know in advance how long an AI strategy will take to plan and implement and what the return on investment will be. This in turn makes it difficult for innovators in organizations to get others on board when it comes to garnering support for AI initiatives.
Of the respondents of the Rackspace survey, 18 percent believed that a lack of clear business case was the main barrier to adopting AI strategies. Lack of commitment from executives was also among the top barriers. Lack of use cases and commitment from senior management show up again among the top challenges in the machine learning journey.
'AI often wanders around as a solution looking for a problem within organizations. I believe this is one of the greatest impediments to its wide-scale adoption within organizations,' DeVerter said. 'As AI practitioners can demonstrate practical examples of how AI can benefit their specific company — leadership will further fund those activities. Like any business venture — leadership needs to know how it will either help them save or make money.'
Evaluating the outcome of AI initiatives is very difficult. According to the survey, the top-two key performance indicators (KPI) for measuring the success of AI initiatives were profit margins and revenue growth. Understandably, this focus on quick profits is partly due to the high costs of AI initiatives. According to the Rackspace survey, organizations spend a yearly average of $1.06 million on AI initiatives.
But while a good AI initiative should result in revenue growth and lower costs, in many cases, the long-term value of machine learning is the development of new use cases and products.
'Short-term financial gains can be myopic if they aren't paired with a long-term strategy that can be funded by those short-term gains,' DeVerter said.
If you're in charge of the AI initiative in your organization, make sure to clearly lay out the use cases, the costs, and the benefits of your AI strategy. Decision-makers should have a clear picture of what their company will be embarking on. They should understand the short-term benefits of investing in AI, but they should also know what they will gain in the long run.
Ben Dickson is a software engineer and the founder of TechTalks. He writes about technology, business, and politics. This post was originally published here as a series exploring the business of artificial intelligence.
This story originally appeared on Bdtechtalks.com. Copyright 2021
VentureBeat
VentureBeat's mission is to be a digital town square for technical decision-makers to gain knowledge about transformative technology and transact.Our site delivers essential information on data technologies and strategies to guide you as you lead your organizations. We invite you to become a member of our community, to access:Dokkan Punch Machine Strategy
- up-to-date information on the subjects of interest to you
- our newsletters
- gated thought-leader content and discounted access to our prized events, such as Transform 2021: Learn More
- networking features, and more